The 2022 Asian Summer School in Econometrics and Statistics Series Courses featured an insightful lecture titled "Data Decomposition Methods and Applications in Econometric Studies," delivered by Professor Shouyang Wang, along with A.P. Wei and Dr. Jiang, on the morning of July 22. The course was moderated by A.P. Lu from Dongbei University of Finance and Economics.
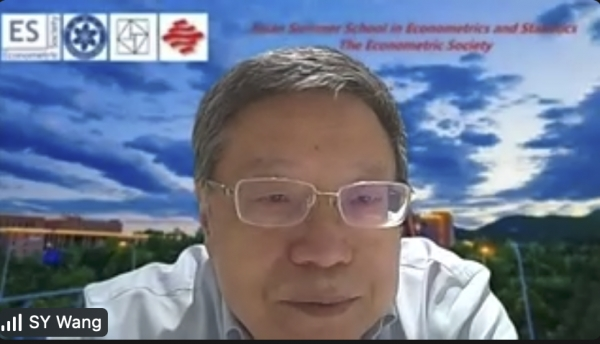
Professor Wang is an Academician of the Academy of Sciences for Developing Countries and International Academy of Systems and Control. He is also a Distinguished Researcher of the Chinese Academy of Sciences, a Distinguished Professor of the Changjiang Scholar Award Program of the Ministry of Education, and the recipient of the National Outstanding Young Scientists Fund. Additionally, he serves as the Director of the Center for Forecasting Science at the Chinese Academy of Sciences and holds editorial roles in over ten domestic and international academic journals. Professor Wang has made significant contributions to decision analysis, supply chain management, risk management, economic analysis and forecasting, epidemic forecasting and policy analysis, earning international acclaim and recognition from relevant governmental decision-making departments.
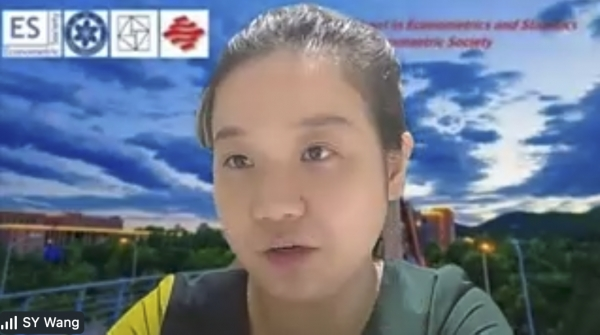
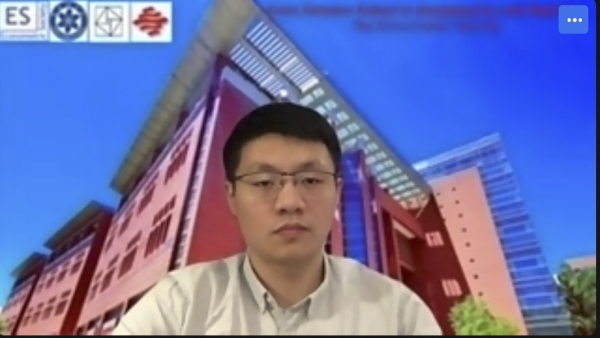
In his lecture, Professor Wang introduced Data Decomposition Methods, which can mitigate the non-linearity and non-stationarity of data. He then presented the Empirical Mode Decomposition (EMD) method proposed by Norden E Huang in 1998, which can be applied directly to non-smooth, non-linear time series. Professor Wang concluded that although there is no complete mathematical theory to support data decomposition methods, these methods have been widely used in the analysis and prediction of complex data, and have achieved numerous successful applications in various fields. He encouraged participants to innovate data decomposition methods, explore applicable methods to specific problems, and combine methods such as EMD with econometric models and deep learning methods to tackle economic and social research challenges.