The 2022 Asian Summer School in Econometrics and Statistics commenced on July 18 with a collaboration between the School of Economics and Management of the University of Chinese Academy of Sciences, Institute of Mathematics and System Sciences of Chinese Academy of Sciences, and Center for Forecasting Science of Chinese Academy of Sciences. Additionally, Paula and Gregory Chow Institute for Studies in Economics of Xiamen University and School of Economics of Dongbei University of Finance and Economics co-organized the event. Esteemed experts in the field of econometrics and statistics were invited to share their knowledge and the latest theoretical frontiers.
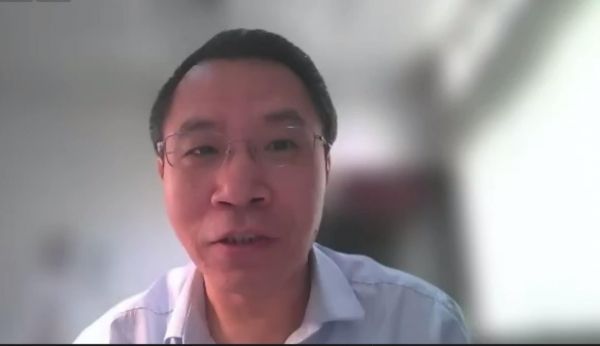
On July 19, Prof. Liangjun Su from Tsinghua University delivered lectures in the morning and afternoon sessions. Prof. Su is a Chair Professor at Tsinghua University, and his research expertise covers non-parametric econometrics, econometric theory, big data analysis, machine learning, factor modeling, and panel data modeling. He has published over 80 research papers in top international journals in information, statistics, and econometrics, including Econometrica, Journal of Applied Econometrics, Journal of Business & Economic Statistics, Journal of Econometrics, Journal of the American Statistical Association, IEEE Transactions on Information Theory, and Quantitative Economics. Prof. Su is also a co-editor of Econometric Theory and an associate editor of Journal of Econometrics and Econometric Reviews.
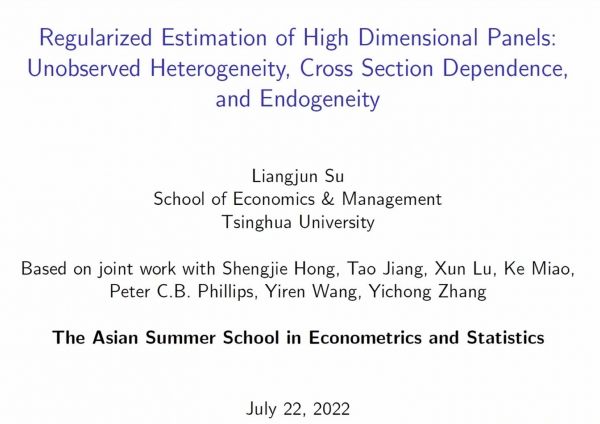
In the morning of July 19, Prof. Su presented his first lecture on "Regularized Estimation of High-Dimensional Panels: Unobserved Heterogeneity, Cross-Section Dependence, and Endogeneity." The lecture was moderated by Ms. Cui from the Department of Economics and Finance at City University of Hong Kong. In the afternoon of July 22, Prof. Su conducted his second course on the same topic, focusing on the estimation and statistical inference of non-linear panel data models, such as high-dimensional VAR models with common factors, panel models with time-varying potential groups, heterogeneous panel quantile models, and potential group identification in network models. Prof. Su emphasized the use of low-rank methods as the common denominator for these models.
During the lecture, Prof. Su introduced the high-dimensional VAR model with common factors in the first part and then taught the panel data model with time-varying potential group structures. Furthermore, he highlighted that quantile regression models have been widely applied in homogeneous panel data models. To provide participants with a better understanding of the topic, Prof. Su explained the differences and connections between network models and panel models and extended the low-rank approach to network models by constructing a logistic undirected network formation model and explaining the model setup and potential difficulties.
The participants were engaged throughout the lecture, and at the end, they asked Prof. Su several questions related to the setting of time-varying potential group models, de-biasing in estimation, the advantages of low-rank methods over C-LASSO and K-means methods in dealing with potential group structures, the relationship between functional coefficient panel models and two-way heterogeneous panels, and possible future research directions. Prof. Su patiently answered each of these questions, concluding an informative and interactive session.