On July 23, Professor Yongmiao Hong delivered a lecture titled "Nonparametric Statistics and Machine Learning" at the 2022 Asian Summer School in Econometrics and Statistics. The session was moderated by Xingbai Xu, an Associate Professor from Xiamen University.
As Executive Director of the Center for Forecasting Science at the Chinese Academy of Sciences and Dean of the School of Economics and Management at the University of Chinese Academy of Sciences, Prof. Hong is an Academician of the Academy of Sciences for Developing Countries, a Fellow of the International Association of Applied Econometrics (IAAE), and a Senior Fellow of The Rimini Centre for Economic Analysis (RCEA). His research focuses on model identification testing, non-linear time series analysis, financial econometrics, and empirical studies of the Chinese economy and financial markets. Prof. Hong's research has been published in top international journals in economics, finance, and statistics, including Econometrica, Journal of Political Economy, Quarterly Journal of Economics, Review of Economic Studies, Review of Economics and Statistics, Annals of Statistics, Journal of the American Statistical Association, Journal of the Royal Statistical Society (Series B), and Review of Financial Studies.
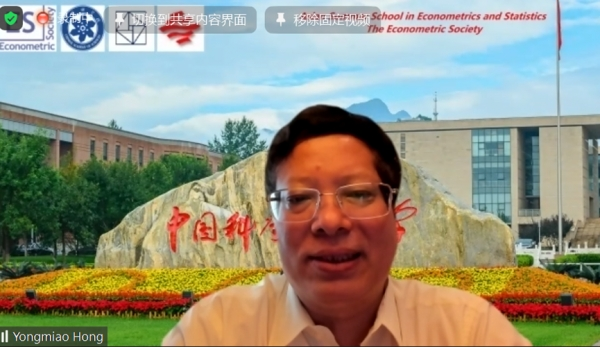
During his lecture, Prof. Hong explained the limitations of traditional parametric methods and emphasized the importance of non-parametric analysis. He introduced the development of non-parametric analysis methods and discussed the two major methods: Global Smoothing and Local Smoothing. Prof. Hong provided examples of global smoothing, such as Sieve Regression and Spline Smoothing, and discussed the trade-offs between Bias and Variance in terms of the smoothing parameter.
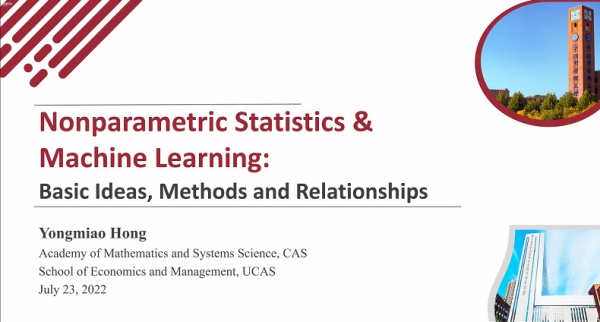
In conclusion, Prof. Hong summarized the methods, relationships, similarities, and differences between Non-parametric Estimation and Machine Learning and answered participants' questions. The lecture was well-received by attendees and concluded the 2022 Asian Summer School in Econometrics and Statistics.